This is a page dedicated to weekly standings for the English Premier League. I am a fan of the Premier League and I am a long time Gooner though try as I might no sort of predictions I do can make them better. The first year was the 2017-18 season for which I did weekly predictions and ended up with 61% accuracy at the end of the year. The second year I got more ambitious and extended the model to include Transfer Market data, and ultimately only made it through 31 weeks.
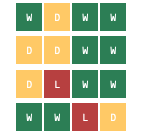